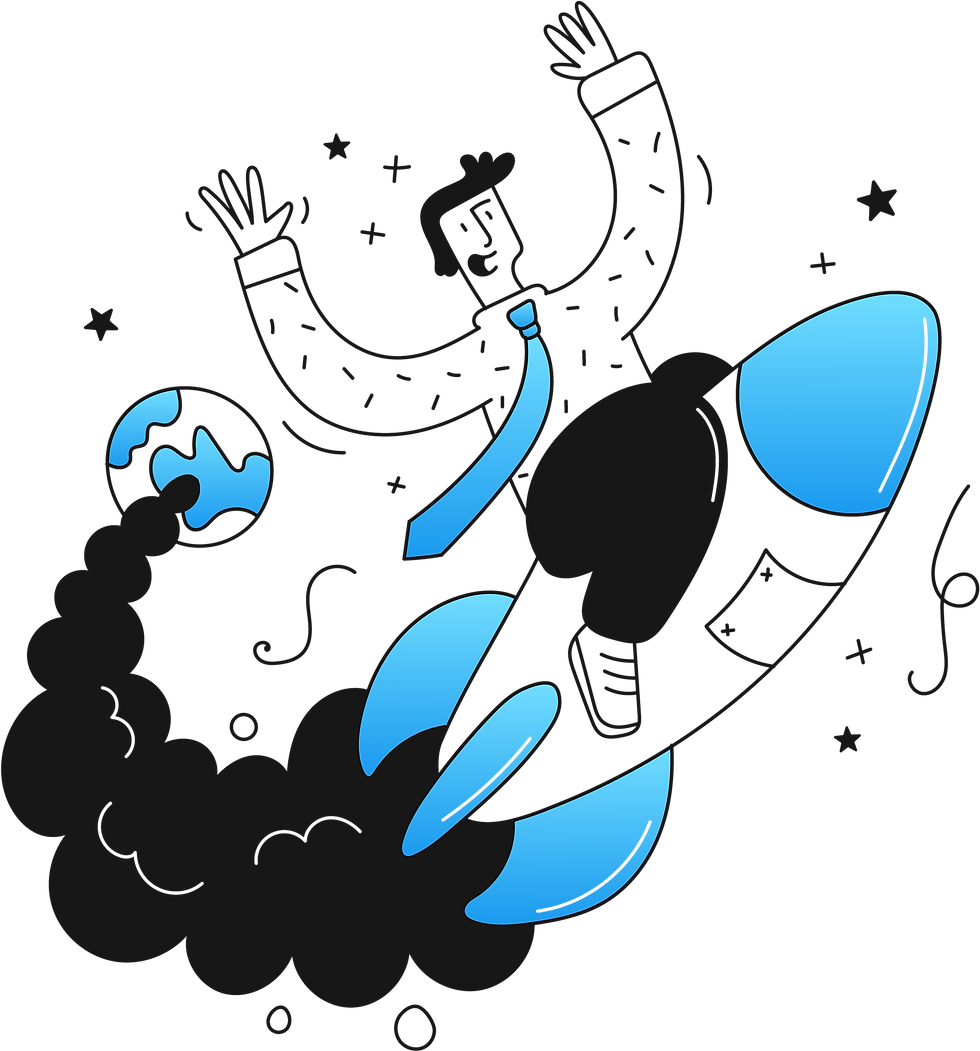
The Jury is Out on Whether AI Will Increase the Number of CNS Drugs on the Market
The process of bringing central nervous system (CNS) disorder treatments to market remains fraught with risks, high costs, and lengthy timelines. Artificial intelligence (AI) technologies promise to address the shortcomings of traditional methods in the drug development process, particularly for CNS therapies. However, the widespread enthusiasm surrounding AI prompts the inevitable question—can AI truly deliver on the potential of bringing more CNS drugs to market, or is it merely hype? This report investigates the impact of AI on advancing the CNS drug development process, exploring groundbreaking advancements, success stories, and essential strategic considerations to foster the growth of this rapidly evolving industry.
Modern CNS Drug Development is an Arduous Journey with High Stakes
The demand for effective CNS disorder treatments has never been higher. Impacting approximately one-third of the U.S. population and standing as the leading cause of disability worldwide, a major biotech investment opportunity presents itself.[1,2] Current treatment options for neurological disorders are few and far between, particularly when one narrows in on only those which alter the course of a disease rather than simply managing symptoms.[1,3] Beneath this unmet need among patients with neurological symptoms lies a therapeutic market poised for substantial growth in the coming years.
Despite the substantial population in need of effective therapies, the CNS drug development process is notoriously challenging, carrying disproportionately high risks compared to therapies in other markets.[4] CNS drugs have a mere 6.2% chance of achieving market access; less than half that of non-CNS drugs (13.3%).[4] CNS drugs also take 31% longer to reach approval than those in other disease areas, extending the average timeline from initial drug discovery to final commercialization stages to 16–20 years.[4] This prolonged development process significantly inflates clinical trial costs, now averaging close to $3.3 billion for a single CNS-targeted product, approximately 30% higher than its non-CNS counterparts.[5] Collectively, developing successful CNS therapies proves to be notably lengthier, costlier, and riskier compared to pharmacological treatments for other diseases.
CNS Complexities Make Drug Development More Challenging in this Arena
Challenges specific to CNS significantly influ[]ence the success of bringing innovative therapies to market. For instance, researchers’ limited understanding of the root causes and mechanisms of CNS diseases complicates the identification of viable drug targets, leading to reliance on brute force trial-and-error approaches.[4,6] This high-risk, resource-intensive process involves systematically testing a vast array of potential drug compounds without precise knowledge of their targets or mechanisms.[4,6]
The use of animal and cell models to test the effectiveness of potential drug targets introduces additional challenges. Disease models are typically designed to mimic specific genetic mutations associated with a condition.[7] However, without a clear understanding of the genetic factors, as is the case for many CNS disorders, accurately replicating the disease in experimental models is practically impossible.[4,7] Even when genetic underpinnings are known, these models usually fall short of capturing the full complexity of CNS disorders.[4,7] As such, imperfect models may prevent accurate predictions as to how potential treatments will perform in clinical settings.[4,7]
Ensuring drug delivery to the brain is another crucial but formidable task for CNS researchers. The blood-brain barrier, a protective shield around the brain, prevents approximately 98% of drugs from reaching their target.[8] Modifying drugs to effectively cross the blood-brain barrier is a complex process that demands expert knowledge, contributing to prolonged development timelines and increased financial investments in specialized drug modification technologies.[8,9]
Clinical stage studies for CNS disorders encounter persistent challenges, such as the absence of accurate biomarkers.[4,10] Biomarkers provide quantifiable metrics of treatment efficacy and safety, leading to efficient trial designs and regulatory approval processes. Therefore, when they are limited, it is all the more challenging to gauge a drug’s effectiveness in clinical studies, increasing the likelihood of trial halts and other such delays.[10,11]
Combined, these challenges extend the drug development timeline, as well as contribute to the overall complexity and resource demands required to bring new therapies to market. Tackling these challenges is critical to transform investments in CNS drug development into a profitable venture. New developments in AI technology promise to address many of these pain points, but the question remains—will it live up to its hype?
AI Has the Potential to Revolutionize the CNS Drug Development Process
AI technologies are poised to revolutionize the entire landscape of CNS drug development.
Data-driven AI methods provide a systematic and efficient alternative to trial-and-error screening and literature reviews. These methods are applicable even to CNS disorders with poorly understood biology or lacking established animal models.
Machine learning, an AI-based approach involving the development of algorithms that enable computers to learn from provided data, has powerful applications in drug target identification. Machine learning models excel at recognizing patterns and correlations within extensive datasets.[12] Such models can integrate information about human genes, proteins, and metabolic processes with patient data to effectively pinpoint novel drug targets.[12,13] Additionally, novel drug targets can be identified by employing natural language processing techniques, which enable AI to parse human language and scan large amounts of scientific literature.[14] These AI-driven approaches eliminate the need for tedious manual literature searches and allow for a more comprehensive analysis of large datasets.[14] This not only speeds up the target identification process, but may also reveal novel targets that may not be readily apparent to human researchers.
Another way machine learning tools could expedite therapy developments is during the formulation process of lead drug candidates. Models can guide researchers in designing compounds with optimal characteristics, making them safer and more effective for human use.[15] Specifically for CNS drugs, machine learning models are capable of predicting a drug's ability to cross the blood-brain barrier based on its biological activities and chemical structures.[16,17] These predictive models streamline drug formulation processes by substantially reducing the experimental testing burden, allowing researchers to focus on the most promising candidates.
The lengthy process of identifying novel targets and optimizing drug formulations can be circumvented almost entirely with drug repurposing, which entails discovering new therapeutic uses for existing drugs.[12,18] AI’s ability to rapidly analyze vast datasets enables it to efficiently pinpoint suitable drugs, outperforming conventional approaches such as literature reviews or chance observations of unintended side effects.[12,18] By leveraging medications already approved for clinical use, AI-powered drug repurposing could significantly cut costs and time involved in preclinical research, as well as reduce regulatory hurdles during clinical trials.[12]
AI’s potential extends to identifying suitable biomarkers for CNS disorders. Machine learning algorithms can analyze large repositories of patient data, such as information about genes and brain scan images, to find patterns that may correlate to disease states.[19] These patterns can serve as biomarkers by indicating the presence or severity of a disease.[19] This capability allows AI to identify biomarkers even in cases where diseases are complex, heterogenous, or have unclear mechanisms, as is often the case for CNS disorders.[19]
All in all, AI boasts a wealth of potential applications that could reshape the field of CNS drug development, ranging from predicting drug targets to enhancing drug repurposing and biomarker identification. However, the true impact of AI can only be gauged through practical implementation and measurable successes in CNS drug development.
Bay Area Startups are Already Reaping the Rewards of AI-Guided CNS Drug Development
While the integration of AI with CNS drug development remains primarily in its early research phases, a handful of companies have boldly transformed these endeavors into tangible achievements. We spotlight two San Francisco Bay Area-based companies that have emerged as trailblazers in leveraging AI to enhance the CNS drug development process.
Verge Genomics, has significantly advanced the preclinical drug development pipeline for neurological diseases using sophisticated machine learning models to identify and develop novel drugs.[20] Their innovative approach involves mining a database of patient genomic data with machine learning models to identify novel genetic drivers of diseases.[20] Subsequently, compounds targeting these genetic drivers are tested in the laboratory. The resulting data serve a dual purpose – evaluating the effectiveness and safety of the drugs while also enriching the dataset used for the machine learning models.[20] This iterative retraining process ensures constant refinement of target identification models. As a proof-of-concept of their pipeline, the startup discovered a novel target and developed it into a clinical candidate within just four years.[20] Their best-in-class drug is a small molecule inhibitor targeting PIKfyve for the treatment of amyotrophic lateral sclerosis (ALS), a neurodegenerative disease characterized by motor neuron loss and paralysis.[20–22] In preclinical studies, the drug effectively reduced motor impairments in a mouse model of ALS.[23] Verge recently reported the completion of a successful Phase Ia clinical trial but later-stage clinical trials are still ongoing.[24] Nonetheless, the application of AI to identify and develop novel targets at unprecedented speeds stands as an impressive feat.
Alto Neuroscience has set an ambitious goal: to develop the first set of personalized biomarkers for the brain.[25] Their approach leverages AI to identify accurate biomarkers for mental health conditions such as post-traumatic stress disorder (PTSD). For instance, they employed a machine learning algorithm to identify signatures from an electroencephalogram (EEG), a noninvasive tool to measure of brain activity. This signature predicts whether a PTSD patient would be resistant to two common treatments.[26] In a preliminary clinical trial, PTSD patients deemed responsive to treatment based on this biomarker exhibited greater improvement in symptoms compared to patients lacking the requisite biomarker signature.[26] Alto Neuroscience’s groundbreaking use of AI to uncover biomarkers predicting treatment responsiveness unlocks the ability to categorize patients based on their expected response to treatments. This breakthrough paves the way for delivering personalized treatments to individual patients based on their unique biology.
While AI-driven drug development for CNS disorders is still in its nascent stages, pioneering companies like Verge Genomics and Alto Neuroscience have already made significant strides, yielding tantalizing and promising results. These compelling examples affirm that AI is already disrupting the CNS drug development landscape, ushering in much-needed therapies to market.
The Bay Area is a Prime Destination for Investors Interested in CNS Drug Development
The successful implementation of AI-driven drug development for CNS disorders hinges on the access to a specialized talent pool proficient in AI, computational biology, and drug development.[27,28]
For investors seeking opportunities in biotech companies within this space, the San Francisco Bay Area emerges as a prime destination for such initiatives. Nestled beside Silicon Valley, the Bay Area offers unparalleled access to a highly skilled workforce. Surrounding world-class universities, such as Stanford, UC San Francisco, and UC Berkeley, bolster innovation through recently established research departments committed to building AI-enabled drug development tools and training the next generation of multidisciplinary scientists. Furthermore, regular collaborative events, bringing together AI and life science experts, foster an environment that breaks down silos between diverse researchers. This rich landscape of talent, partnerships, and initiatives positions the San Francisco Bay Area as an optimal destination for investors seeking to capitalize on the future of AI-assisted CNS drug development.
AI-Enabled Drug Development Will Inevitably Bring More Novel CNS Drugs to Market
The growing prevalence of CNS disorders, coupled with limited treatment options, signifies an expanding market for CNS drugs. However, the conventional methods of CNS drug development are notoriously risky, costly, and time-consuming. AI-powered technologies are poised to revamp these processes and bring much needed CNS drugs to market. Pioneering startups, like Verge Genomics and Alto Neuroscience, have already made promising strides applying AI technologies to expedite the arrival of CNS drugs to market, supporting the credibility of AI in drug development. Access to a particular and skilled talent pool is needed to keep up with the increasing demands of AI-led drug development technologies. Situated at the intersection of cutting-edge technology, top-tier talents, and a regional commitment to transformative healthcare solutions, the Bay Area is uniquely positioned to meet such demands.
Now is the time to invest in AI-powered drug discovery. Unlock unprecedented capital gains in CNS therapeutic landscapes by tapping into the plethora of talent and exceptional potential in the San Francisco Bay Area.
References
1. Owolabi MO, Leonardi M, Bassetti C, et al. Global synergistic actions to improve brain health for human development. Nat Rev Neurol. 2023;19(6):371-383. doi:10.1038/s41582-023-00808-z
2. Feigin VL, Nichols E, Alam T, et al. Global, regional, and national burden of neurological disorders, 1990–2016: a systematic analysis for the Global Burden of Disease Study 2016. Lancet Neurol. 2019;18(5):459-480. doi:10.1016/S1474-4422(18)30499-X
3. Carroll WM. The global burden of neurological disorders. Lancet Neurol. 2019;18(5):418-419. doi:10.1016/S1474-4422(19)30029-8
4. Gribkoff VK, Kaczmarek LK. The Need for New Approaches in CNS Drug Discovery: Why Drugs Have Failed, and What Can Be Done to Improve Outcomes. Neuropharmacology. 2017;120:11-19. doi:10.1016/j.neuropharm.2016.03.021
5. DiMasi JA, Grabowski HG, Hansen RW. Innovation in the pharmaceutical industry: New estimates of R&D costs. J Health Econ. 2016;47:20-33. doi:10.1016/j.jhealeco.2016.01.012
6. Dreiman GHS, Bictash M, Fish PV, Griffin L, Svensson F. Changing the HTS Paradigm: AI-Driven Iterative Screening for Hit Finding. Slas Discov. 2021;26(2):257-262. doi:10.1177/2472555220949495
7. Chesselet MF, Carmichael ST. Animal Models of Neurological Disorders. Neurotherapeutics. 2012;9(2):241-244. doi:10.1007/s13311-012-0118-9
8. Pardridge WM. Blood-brain barrier delivery. Drug Discov Today. 2007;12(1-2):54-61. doi:10.1016/j.drudis.2006.10.013
9. Morofuji Y, Nakagawa S. Drug Development for Central Nervous System Diseases Using In vitro Blood-brain Barrier Models and Drug Repositioning. Curr Pharm Des. 2020;26(13):1466-1485. doi:10.2174/1381612826666200224112534
10. Ward M, Schofield EL. Biomarkers for brain disorders. Therapy. 2010;7(4):321-336. doi:10.2217/thy.10.31
11. Lleó A. Biomarkers in neurological disorders: a fast-growing market. Brain Commun. 2021;3(2):fcab086. doi:10.1093/braincomms/fcab086
12. Paul D, Sanap G, Shenoy S, Kalyane D, Kalia K, Tekade RK. Artificial intelligence in drug discovery and development. Drug Discov Today. 2021;26(1):80-93. doi:10.1016/j.drudis.2020.10.010
13. Zhavoronkov A, Vanhaelen Q, Oprea TI. Will Artificial Intelligence for Drug Discovery Impact Clinical Pharmacology? Clin Pharmacol Ther. 2020;107(4):780-785. doi:10.1002/cpt.1795
14. Bhatnagar R, Sardar S, Beheshti M, Podichetty JT. How can natural language processing help model informed drug development?: a review. JAMIA Open. 2022;5(2):ooac043. doi:10.1093/jamiaopen/ooac043
15. Han R, Yoon H, Kim G, Lee H, Lee Y. Revolutionizing Medicinal Chemistry: The Application of Artificial Intelligence (AI) in Early Drug Discovery. Pharmaceuticals. 2023;16(9):1259. doi:10.3390/ph16091259
16. Kumar R, Sharma A, Alexiou A, Bilgrami AL, Kamal MA, Ashraf GM. DeePred-BBB: A Blood Brain Barrier Permeability Prediction Model With Improved Accuracy. Front Neurosci. 2022;16. Accessed February 27, 2024. https://www.frontiersin.org/journals/neuroscience/articles/10.3389/fnins.2022.858126
17. Notwell JH, Wood MW. ADMET property prediction through combinations of molecular fingerprints. Published online September 29, 2023. Accessed February 27, 2024. http://arxiv.org/abs/2310.00174
18. Ahmed F, Soomro AM, Chethikkattuveli Salih AR, et al. A comprehensive review of artificial intelligence and network based approaches to drug repurposing in Covid-19. Biomed Pharmacother. 2022;153:113350. doi:10.1016/j.biopha.2022.113350
19. Winchester LM, Harshfield EL, Shi L, et al. Artificial intelligence for biomarker discovery in Alzheimer’s disease and dementia. Alzheimers Dement. 2023;19(12):5860-5871. doi:10.1002/alz.13390
20. Verge Genomics. Pipeline. Accessed February 27, 2024. https://www.vergegenomics.com/pipeline
21. Mejzini R, Flynn LL, Pitout IL, Fletcher S, Wilton SD, Akkari PA. ALS Genetics, Mechanisms, and Therapeutics: Where Are We Now? Front Neurosci. 2019;13:1310. doi:10.3389/fnins.2019.01310
22. Shi Y, Lin S, Staats KA, et al. Haploinsufficiency Leads to Neurodegeneration in C9ORF72 ALS/FTD Human Induced Motor Neurons. Nat Med. 2018;24(3):313-325. doi:10.1038/nm.4490
23. CollisionConf 2019 Healthtech: Verge Genomics - YouTube. Accessed February 27, 2024. https://www.youtube.com/
24. Verge Genomics Announces Positive Safety and Tolerability Data from the Phase 1 Clinical Trial of VRG50635, a Potential Best-in-Class Therapeutic for All Forms of ALS. Verge Genomics. Published June 15, 2023. Accessed February 27, 2024. https://www.vergegenomics.com/news-blog/verge-genomics-announces-positive-safety-and-tolerability-data-from-the-phase-1-clinical-trial-of-vrg50635-a-potential-best-in-class-therapeutic-for-all-forms-of-als
25. Alto Neuroscience. Platform. Accessed February 27, 2024. https://www.altoneuroscience.com/platform
26. Zhang Y, Naparstek S, Gordon J, et al. Machine learning-based identification of a psychotherapy-predictive electroencephalographic signature in PTSD. Nat Ment Health. 2023;1(4):284-294. doi:10.1038/s44220-023-00049-5
27. Fultinavičiūtė U. AI in biotech: Still in search of desired talent and filling the regulatory gap. Clinical Trials Arena. Published October 30, 2023. Accessed February 27, 2024. https://www.clinicaltrialsarena.com/features/ai-biotech-talent-regulation/
28. 2023 State of Tech in Biopharma Report. Accessed February 27, 2024. https://www.benchling.com/state-of-tech
Kommentare